Unlocking Business Potential with Generative AI: Learn how to develop a winning strategy, harnessing the power of Generative AI for content creation, customer support, and innovation. Navigate data quality, model selection, ethics, and ROI to stay at the forefront of technological transformation.
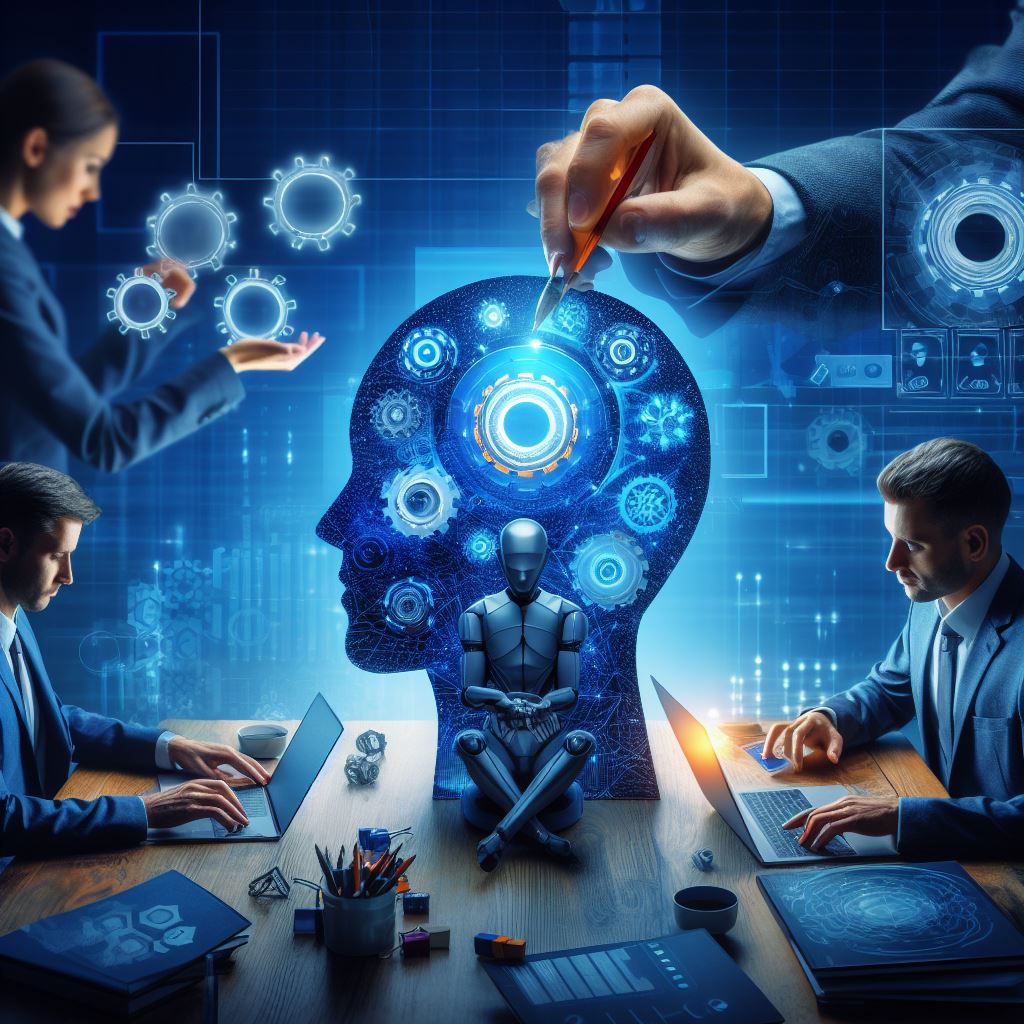
Introduction
In today’s ever-evolving digital landscape, the relentless march of technology has become the ultimate differentiator in the business world. Among the many technological marvels, Generative AI stands out as a potential game-changer. Capable of transforming the way businesses operate and communicate, Generative AI models like GPT-3 have left an indelible mark on various industries. To harness this transformative power, businesses must formulate a unique and tailored Generative AI strategy. In this article, we will explore the distinct steps to develop a Generative AI strategy that sets your business on the path to success.
Understanding the Essence of Generative AI
Before diving into strategy development, it is paramount to grasp the essence of Generative AI and its boundless potential. Generative AI is a facet of artificial intelligence that revolves around generating content, data, or even software by comprehending underlying patterns. These deep learning models, often trained on vast datasets, can create human-like text, images, code, and more.
From content generation and chatbots to data analysis and process automation, Generative AI offers a myriad of applications that can save time, enhance efficiency, and elevate customer experiences.
Setting Clear Business Objectives
The foundational stage of crafting a Generative AI strategy involves setting crystal-clear business objectives. What specific challenges or opportunities does your business confront, which Generative AI can elegantly address? These objectives must be specific, measurable, and align seamlessly with your broader business goals. For instance:
- Content Creation: Can Generative AI be employed to automate content generation for your website or social media platforms?
- Customer Support: Is there a need to enhance your customer support with AI-driven chatbots?
- Data Analysis: Can Generative AI assist in deciphering large datasets to unearth valuable insights?
- Innovation: Is Generative AI instrumental in generating novel product ideas or prototypes?
- Process Automation: How can Generative AI streamline internal processes, fostering efficiency?
- Cost Reduction: Is it possible to leverage Generative AI to trim operational costs?
These well-defined objectives form the bedrock of your Generative AI strategy, serving as guiding stars for your business’s AI journey.
Prudent Data Collection and Preparation
Generative AI is data-hungry, and the quality of the data you feed into the system is the key to success. For text generation, pristine and structured text data is essential, while image generation requires an extensive and relevant image dataset.
Moreover, the data should be a mirror image of your business domain. For e-commerce, it must be replete with product information, customer reviews, and related content. In healthcare, medical records and relevant documents should take center stage.
Crafting a Generative AI strategy necessitates the arduous task of curating and preprocessing data. Although it can be resource-intensive, the significance of data quality cannot be overstated.
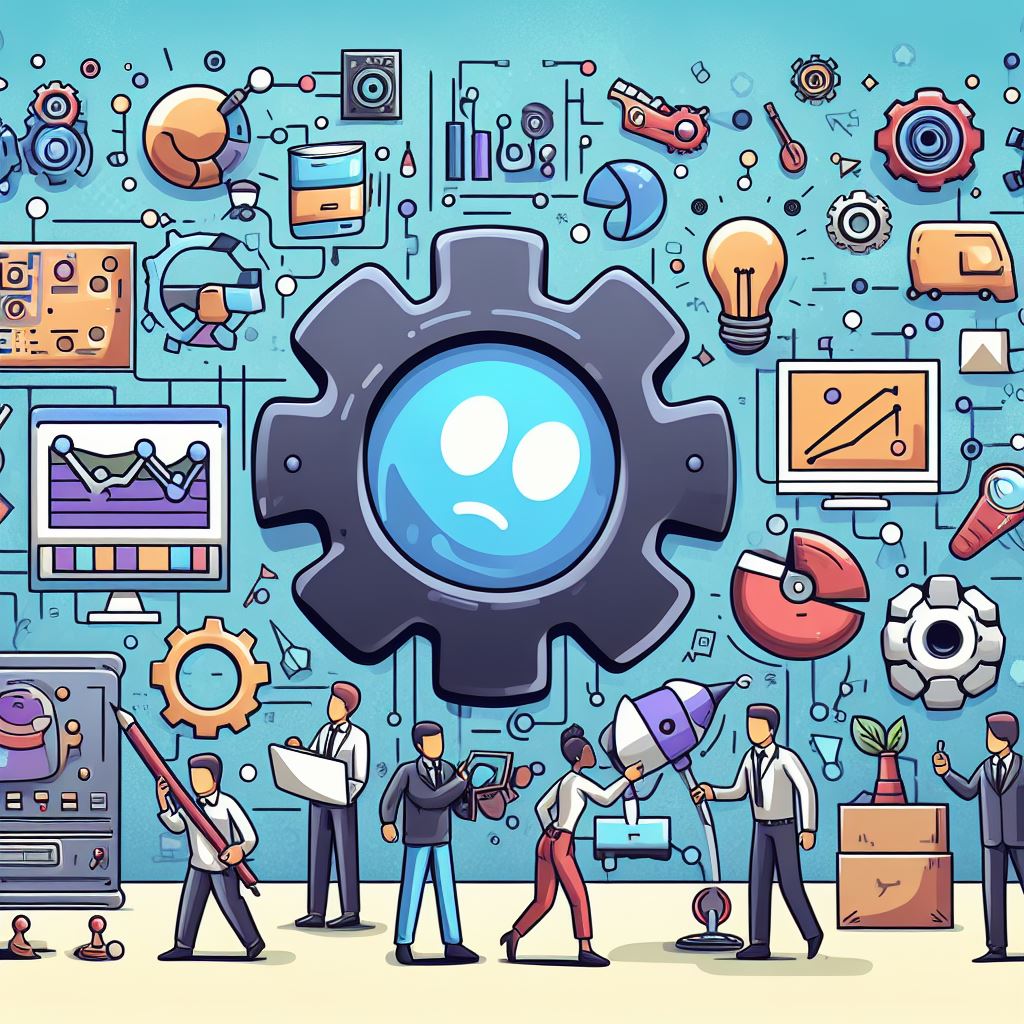
The Art of Model Selection
Selecting the right model is a pivotal decision within your Generative AI strategy. GPT-4 is a front-runner. Nonetheless, the Generative AI arena is a hotbed of innovation, and new models are emerging soon.
When it comes to model selection, considerations like model size, performance, scalability, and ethical implications are paramount. Smaller models can be cost-effective and efficient, while GPT-4 and its peers bring phenomenal capabilities but often at a higher cost.
Furthermore, ethics loom large in this domain. Generative AI models can inadvertently spawn biased or undesirable content. You must impress upon your readers the indispensability of ethical AI usage, underpinned by guidelines and policies, to ensure responsible AI deployment.
The Delicate Art of Training and Fine-Tuning
After selecting the model, the stage is set for training and fine-tuning. Training entails exposing the model to your data, allowing it to decipher the patterns and nuances contained within.
Fine-tuning is the secret sauce that makes the model tailor-made for your business’s unique requirements. If, for instance, you’re crafting a chatbot for a financial application, the model needs fine-tuning to understand the intricacies of financial terminology, regulatory standards, and customer interactions.
This phase is an intricate ballet, and technical expertise is often indispensable. Many businesses resort to data scientists or AI experts to ensure the model is trained and fine-tuned impeccably.
Implementation and Assimilation
Once your model is finely tuned, it’s time to unleash it into your business processes. The mode of implementation can be vastly diverse, contingent upon your objectives. This might entail embedding AI into your website, mobile app, or customer service systems. The seamless integration of AI should be presented to your readers as the lynchpin of a successful Generative AI deployment.
The Crucial Phase of Testing and Quality Assurance
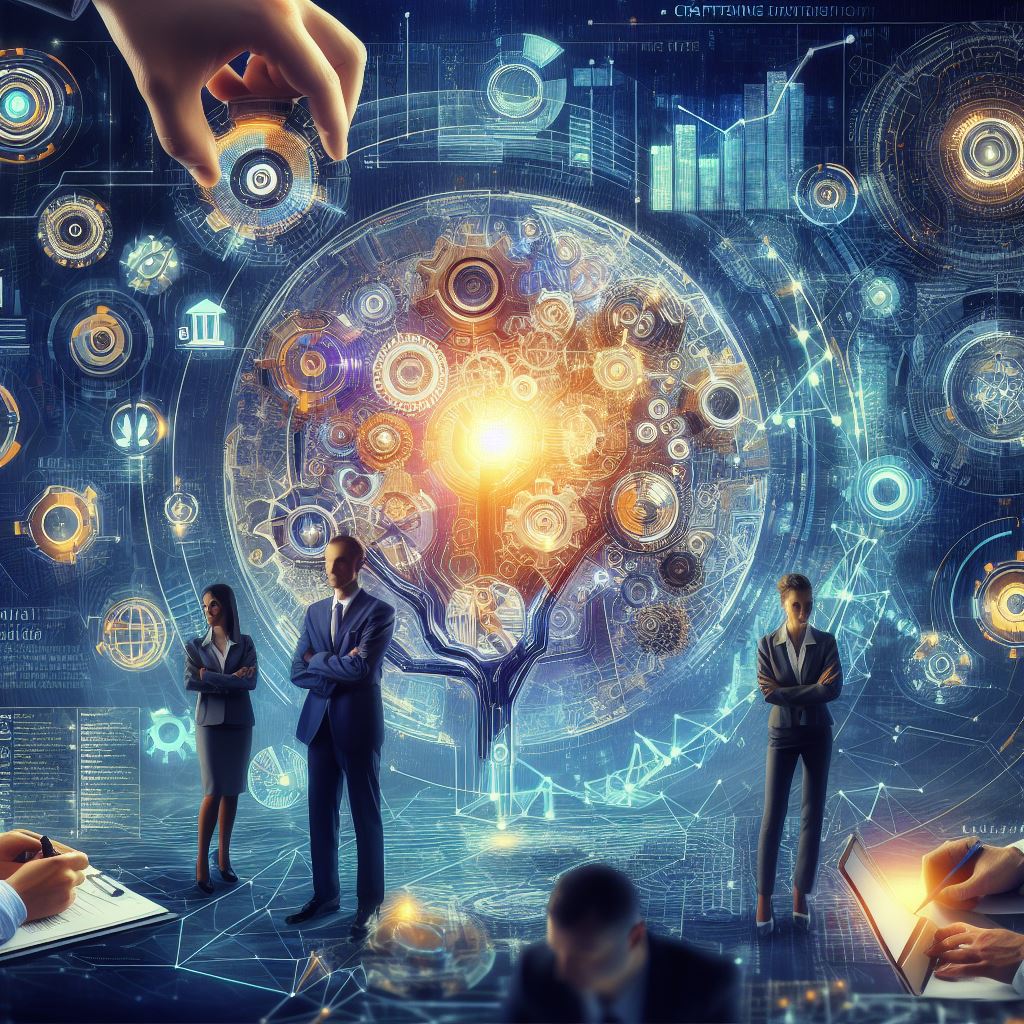
Before your Generative AI solution sees the light of day, rigorous testing and quality assurance are non-negotiable. This phase guarantees that the AI behaves as expected, generates accurate and meaningful content, and avoids the creation of biased or harmful output. Impress upon your readers the iterative nature of this process, often necessitating multiple rounds of testing and refinement.
The Imperative of Monitoring and Maintenance
Generative AI is not a one-and-done affair. It demands consistent monitoring and maintenance to ensure that the model maintains its accuracy and relevancy. Models can become outdated or less accurate over time, necessitating regular updates and retraining.
Furthermore, vigilant monitoring is critical to the early detection and mitigation of ethical concerns or biases that may crop up in AI-generated content.
The Inviolable Mandate of Data Security and Privacy
Incorporating Generative AI in a business context places data security and privacy at the forefront. Your readers must be acutely aware of the need to safeguard customer data and ensure that their AI strategy complies with relevant data protection regulations.
The Moral Compass: Ethical Considerations
Generative AI can, on occasion, yield content that is offensive, biased, or misleading. In your capacity as a tech and business journalist, you must vociferously underline the critical significance of ethical guidelines, content moderation, and the responsible use of AI to preclude the proliferation of harmful content.
The Ledger of Cost Analysis
Crafting a Generative AI strategy entails an investment, and a meticulous cost analysis is obligatory. This analysis encompasses data acquisition, model selection, training and fine-tuning, integration, testing, and the perpetual maintenance of the AI solution. The return on investment (ROI) should also be scrutinized, considering the impact on productivity, customer satisfaction, and other key performance indicators.
The Unceasing Pursuit of ROI Evaluation
Following the implementation of the Generative AI strategy, an unceasing evaluation of its ROI is nonpareil. Are the objectives being met, and is the AI strategy yielding value to the business? Assess the ramifications on productivity, customer satisfaction, and other key performance indicators.
The Pivotal Path of Scaling and Growth
As businesses evolve, their Generative AI strategy must evolve in tandem. Your readers should be acutely aware of the importance of flexibility within their AI strategy, permitting them to adapt to future growth and shifting objectives.
In Summation
Generative AI is an unstoppable force, poised to redefine the landscape of business operations and customer engagement. Crafting a successful Generative AI strategy demands a meticulous approach, characterized by clearly defined objectives, pristine data, judicious model selection, impeccable training and fine-tuning, seamless integration, and ongoing monitoring and maintenance.
Leave a Reply Cancel reply